Research Interests
- Medical Video Analysis
- Multimedia Retrieval
- Interactive Multimedia
- Multimedia Exploration
- Applied Machine Learning
Current Research Projects
SQUASH – Surgical Quality Assessment in Gynecologic Laparoscopy
Funded by: FWF Austrian Science Fund under grant P 32010-N38
Duration: April 2019 – September 2024

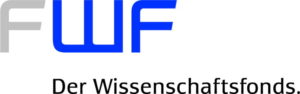
Endoscopic surgeries require specific psychomotor skills that are difficult to learn and teach, and typically result in prolonged learning curves. These psychomotor skills have direct impact on the performance of the surgery, especially in a field with complex operation techniques. In order to assess surgical quality objectively, medical experts currently record the entire surgery on video and inspect and analyze the unedited video footage in a post-operative session for the occurrence of technical errors, according to some standardized rating scheme. Several studies have shown that such post-operative analysis of errors and the reporting of them to the responsible surgeons can significantly improve their performance over time and lead to better surgical quality, especially for young surgeons. However, currently the surgical quality assessment (SQA) process is so tedious and time-consuming that many surgeons/clinicians cannot afford to perform such error ratings, which is very unfortunate since their application would improve surgical quality and patient outcome. The main reason for the high effort is the fact that it is performed without any special error rating software, but with common software video players and manually edited checklists, where surgeons enter timestamps of corresponding relevant scenes in the video. This renders SQA currently not only a very time-consuming process, but also a very error-prone one.
In this research project we want to address this issue and find out how we can make surgical quality assessment (SQA) more efficient through automatic video content analysis and, hence, more feasible. More specifically, for the field of gynecologic laparoscopy we want to investigate to what extent current methods of machine learning and content-based video retrieval can support SQA (i.e., optimize the entire process through automatic classification and retrieval of technical errors). For that purpose, we will evaluate deep learning approaches as well as video content description and similarity search.
OVID: Relevance Detection in Ophthalmic Surgery Videos
Funded by: FWF Austrian Science Fund under grant P 31486-N31
Duration: October 2018 – September 2022
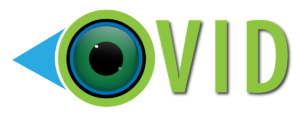
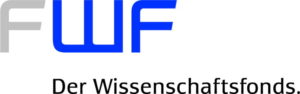
In this project, we want to investigate fundamental research questions in the field of postoperative analysis of ophthalmic surgery videos (OSVs). More precisely, three research objectives are covered: (1) Classification of OSV segments – is it possible to improve upon the state-of-the-art in automatic content classification and content segmentation of OSVs, focusing on regular and irregular operation phases? (2) Relevance prediction and relevance-driven compression – how accurately can the relevance of OSV segments be determined automatically for educational, scientific, and documentary purposes (as medical experts would do), and what compression efficiency can be achieved for OSVs when considering relevance as an additional modality? (3) Analysis of common irregularities in OSVs for medical research – we address three quantitative medical research questions related to cataract surgeries, such as: is there a statistically significant difference in duration or complication rate between cataract surgeries showing intraoperative pupil reactions and those showing no such pupil reactions?
Finished Research Projects
- 2015-2019: Knowledge & Information Sharing in Medical Expert Teams (KISMET), Lakeside Labs Research Project (Principal Investigator)
- 2015-2018: Endoscopic Video Processing 2 (EndoVIP2), Contract Research Project (Principal Investigator)
- 2012-2015: Endoscopic Video Processing (EndoVIP), Contract Research Project (Principal Investigator)
- 2012-2015: Community of Domain Experts in Medical Multimedia (CODE-MM), Lakeside Labs Research Project KWF-20214 22573 33955 (Co-Principal Investigator)
- 2012-2016: Next-Generation Video Browsing (NGVB), FWF Translational Research Project TRP 273-N15 (Principal Investigator)